7 Reasons Why Machine Learning is Crucial for Business
In the midst of the rapid development of artificial technology or Artificial Intelligence (AI), not many people realize that true artificial intelligence consists of several branches, one of which is machine learning. Machine learning is one of the branches of AI that is improving for companies. As we know before, machine learning is a machine that can learn like a human.
Curious about how machine learning works and how it benefits businesses? Find out more about machine learning complete with real-world benefits in this article!
What is Machine Learning?
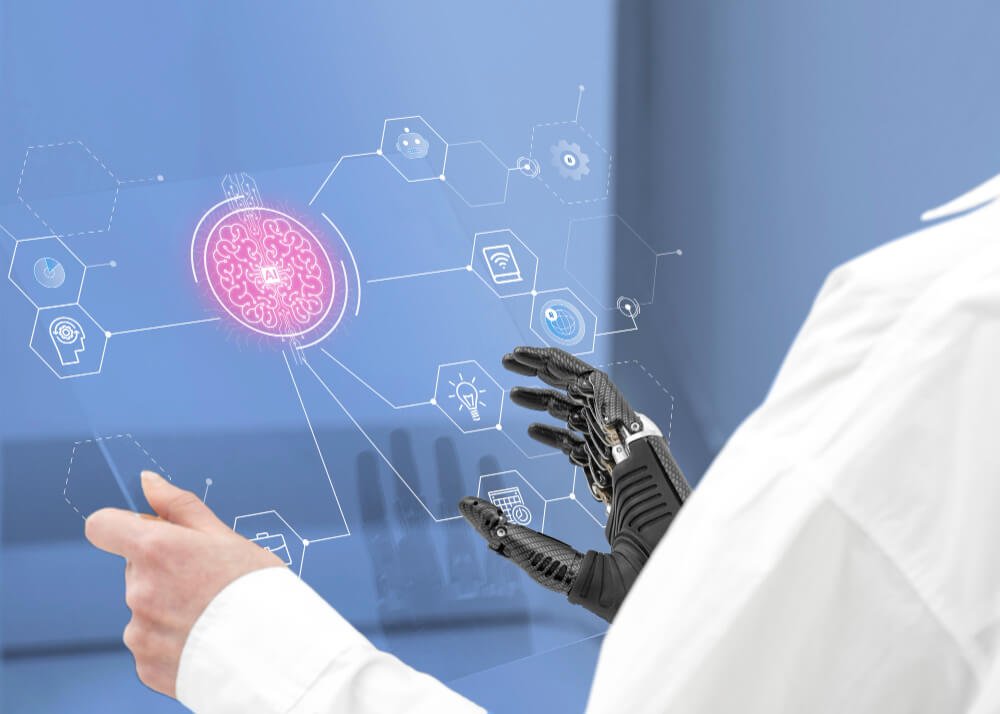
Machine learning is artificial intelligence and computer science that focuses on using data management and algorithms to mimic the way humans learn. From this process, the machine will learn gradually to improve its accuracy. In this context, machine learning will focus on developing computer programs that can access data and use it to learn on their own.
The term machine learning first came from several mathematical scientists such as Adrien Merie Ledenre, Thomas Bayes, and Andrey Markov. In the 1920s, they tried to describe the basics of machine learning and its concepts.
Since then, many companies have tried to develop machine learning. One of the most famous applications of machine learning technology was IBM’s Deep Blue in 1996.
How Does Machine Learning Work?
In the current era, the role of machine learning can help humans in various fields. Even its application can be easily found in everyday life. For example, when using the face unlock feature to open a smartphone, or when browsing the internet and finding ads on social media. This section will explain how machine learning works so that it can be useful for business activities.
Difference Between Artificial Intelligence and ML
Most people, especially those who are beginners to machine learning and artificial intelligence, think that both of them are the same thing. In fact, they are quite different. To facilitate understanding, let’s understand the definition of AI first.
Artificial Intelligence is a technology used to develop computers and robots that can behave like humans, or even exceed human needs. The programs behind AI can analyze and contextualize data so the machines can perform actions automatically based on the information obtained.
Today, AI has been adopted by a series of technologies, such as virtual assistants including Google Assistant, Siri, and others. In the business field, companies are combining techniques such as Natural Processing Language and Computer Vision to automate tasks for customer experience optimization. An example is two-way conversations that can be conducted at any time between customers and businesses through chatbots.
As mentioned earlier, machine learning basically is a branch of AI. In Machine Learning, computers will learn to extract patterns from data visualization using training data. The results of these activities can be used to make decisions. However, before being able to use the trained model to make decisions, of course, the model must be tested. As accuracy increases, the model’s ability to generalize the available data will also increase
Machine Learning is closely related to AI, so it is not surprising that a lot of people think these two are similar things. Machine Learning itself plays an important role in AI. Even if it is likened, Machine Learning is the brain of humans while AI is the whole head. But, in fact, AI can still work without involving Machine Learning.
3 Categories of Machine Learning Methods
In its development, machine learning is carried out with three main methods:
- Supervised Learning
Supervised learning method is done by labeling the dataset used by machine learning and classified by the developer by allowing the algorithm to see the accuracy of its performance.
The way this method works is by inputting information as input and labeled data as result or output. The supervision of machine learning in this method is done by the labeled data which in turn makes machine learning learn what the relationships and dependencies are between the data.
- Unsupervised Learning
This method is also known as semi-supervised learning, where it does not require human assistance for the computer to actually learn the data and its relationships on its own.
In this case, the dataset is unlabeled and the machine computationally identifies patterns in the data. Unsupervised learning methods are used to make it easier for developers to make decisions.
- Reinforced Learning
Reinforced learning methods operate by using reward/punishment datasets and offering feedback to the algorithm to learn from ‘random’ experiences.
This ‘random’ method is similar to the pattern understanding system that humans use when learning something from experiments.
6 Commonly Used Machine Learning Algorithms
When exploring the world of machine learning, you are required to be familiar with the various types of algorithms that exist:
- Decision Tree
Decision tree is a type of algorithm that is relatively easy to understand and intuitive. It uses a tree-like model and then describes the possible outcomes. It is usually used for classification and regression tasks.
- Linear Regression
Linear regression is a basic algorithm that helps to understand the relationship between two variables. It is often used to guess the value of a number or to provide an understanding of the influence of various factors on the target variable.
- Logistic Regression
Similar to linear regression, logistic regression is used to guess the outcome in solving binary classification problems. This algorithm is often used for various fields such as medical diagnosis, credit scoring, and spam detection.
- Random Forest
This type of algorithm combines multiple decision trees to give an overview of the results. This increases the accuracy and reduces overfitting compared to a single decision tree. Usually, random forest is often used to solve classification and regression.
- Naive Bayes
Naive Bayes is a probabilistic algorithm based on Bayes’ theorem, known for its simplicity and efficiency in classifying text and categorical data. This algorithm is often used for sentiment analysis, spam filtering, and document classification.
- Artificial Neural Networks (ANN)
ANN is a class of algorithms inspired by the structure of the human oak nerve. ANNs are commonly used to solve complex tasks such as image recognition, natural language processing, and speech recognition.
6 Real-world Benefits of Machine Learning for Business
The adoption of technology has transformed businesses into a different stone. Talking about the benefits in general, of course, it cannot be separated from the ability of machine learning to develop and grow.
The benefits of machine learning can be obtained quickly and are directly proportional to the amount of what data warehouse (data lake) owned. Here are the benefits that can be felt when applying machine learning:
- Enhance company development
The ability to store and learn information systematically can stimulate companies to develop, grow, or improve over time. The more data that is processed, the more results, variations, and insights that are provided as a reference for decision-making.
Utilizing machine learning, companies not only get results but also analyze to determine other possibilities.
- Resolve problems and obstacles
Companies always face various problems and obstacles in their activities. Information about problems can be processed through machine learning so that it can help companies identify problems from experience without the need for excessive intervention.
Enough input, then machine learning will learn on its own. This method is widely embedded as technology in various computer-based tools.
- Flexible for various industry sectors
The term machine learning seems very technological and many people think that Machine Learning can only be applied by start-ups. This is clearly wrong.
Machine learning can be used by various industrial sectors. In fact, one of its implementations has been carried out on a non-profit project from the United Nations High Commissioner for Refugees (UNHCR) to map refugee camps from Burundi, Rwanda, and Congo.
- Able to store a lot of information
The information that can be stored by machine learning has almost no limit as long as the server or information storage media is still available.
Therefore, a lot of data and information can be stored by machine learning. Of course, this information and data are very useful for business development and growth.
- Predicting Trends and Innovations
Machine learning can forecast trends and innovations according to company characteristics. By processing existing data, companies can find out future predictions. Then, based on these guidelines, the company will make certain plans.
This is very helpful for companies to stay up to date with the times so that the business can be sustainable.
- Improve Personalization and recommendations for customers
Another example of the application of Machine Learning is personalized content such as product recommendations in online shopping systems. Where one of the resources is obtained from search history.
Personalization like this is now a trick to increase customer interest in shopping and increase customer bucket size.
4 Biggest Challenges of Machine Learning
Besides the convenience and sophistication offered, Machine Learning certainly has some challenges that need to consider:
- Models that require testing
Businesses face the challenge of not having adequate infrastructure for modeling. The right infrastructure can help with testing and with the use of various tools. The more frequent testing is done on machine learning models, the more accurate the results or the trained learner will be.
- Concerns related to data security
The other most common challenge is data availability. The availability of raw data is crucial for businesses to deploy machine learning. Because large amounts of data are used to train machine learning algorithms.
Not only that, data security concerns are one of the problems faced in implementing machine learning. Security becomes a very important and highly guarded aspect, where the application of machine learning must distinguish sensitive data and insensitive data for the reason of protecting consumer data.
- Time-consuming
To get maximum results, Machine Learning requires some time. Moreover, Machine Learning must pass various tests so that it can work more optimally and accurately.
In addition, considering that the development will continue, various new trends will emerge, and the demanded business must be able to keep up with these trends. However, sometimes, the application is full of uncertainties and even takes a long time to get accurate machine learning results.
- Lack of knowledge and talents
The most worrying challenge is the limited domain knowledge, which is one of the most important knowledge in machine learning. This is followed by the lack of talents who master machine learning skills.
Although the interest in learning is increasing, it will take time to find a balance between the number of professional machine learning practitioners and the demand in the industry.
Choose the Trusted AI Platform with IBM!
Through this article, we have seen and known how machine learning is being utilized in various industries in many ways. From helping to identify risks, to providing personalized services.
Through the insights provided by machine learning, companies can customize marketing efforts, provide better services for customers, and provide a more personalized experience to build engagement. This will help build loyal audiences and trust in your business.
If you want to explore the tremendous potential of machine learning for your business, IBM Watsonx AI will be the perfect match. With IBM Watsonx AI, businesses can leverage techniques to gain deep insights from large volumes of data, improve operational efficiency, and make better decisions contextually.
Partnering with Optus, as a distributor of IBM products, provides additional support and services that enhance your experience in adopting machine learning for business growth.
Integrating machine learning into business operations through IBM Watsonx AI not only opens the door to greater efficiency but also opens new opportunities for growth and innovation. In addition, as an authorized IBM Watsonx AI distributor, Optus will provide full and exclusive access to the platform.